In medical imaging, the process of creating 3D images is crucial for detailed anatomical analysis and is important for diagnosis. However, creating trustworthy and reliable 3D images is a complex task that involves a number of challenges. This article explores the challenges associated with 3D reconstruction in medical imaging and lists the key factors that influence it.
Zoe Amin-Akhlaghi, ZAMSTEC Academy of Science and Technology, Austria
Table of Contents
Introduction
The development of three-dimensional (3D) medical imaging has ushered in a revolutionary period for the identification and planning of medical treatments. 3D imaging has emerged as a vital tool in modern medicine because it allows physicians to see intricate structures in a manner that is not possible with two-dimensional images. The method of creating these 3D pictures from medical data, or 3D reconstruction, comes with technical difficulties despite its broad applicability. In order to fully realize the promise of 3D imaging technology, it is important to address the issues raised in this article.
The complicated nature of the data itself is at the heart of these challenges. Medical imaging data is inherently complex, this complexity often necessitates the use highly sophisticated software and hardware to both acquire and recreate the data in three dimensions. The precision and usefulness of the reconstructed images are directly impacted by the quality of this data, which can be impacted by a variety of variables such as the movement of patients and the limitations of imaging equipment. thereby, improving 3D reconstruction requires addressing the factors which impact data quality.
Apart from the complicated nature of data acquisition, the steps involved in 3D reconstruction—segmentation, alignment, and rendering—come with their own unique challenges. These procedures depend on how precisely algorithmic functions are applied to the data, which needs to be carefully calibrated in order to yield trustworthy and accurate images. Any miscalculation or neglect at these phases could produce images that are not optimal for clinical usage, which could lead to incorrect diagnoses and treatment plans.
This article’s goal is to examine these difficulties’ practical implications in clinical practice in addition to discussing their technical features. Through an analysis of the challenges faced by technologists and clinicians in 3D medical imaging reconstruction, we hope to bring about a better understanding of these challenges and a conversation that may result in innovative approaches and further advancements in the field. The pathway to better 3D imaging is one that necessitates sustained collaboration along with innovation as we dig further into the details of these challenges.
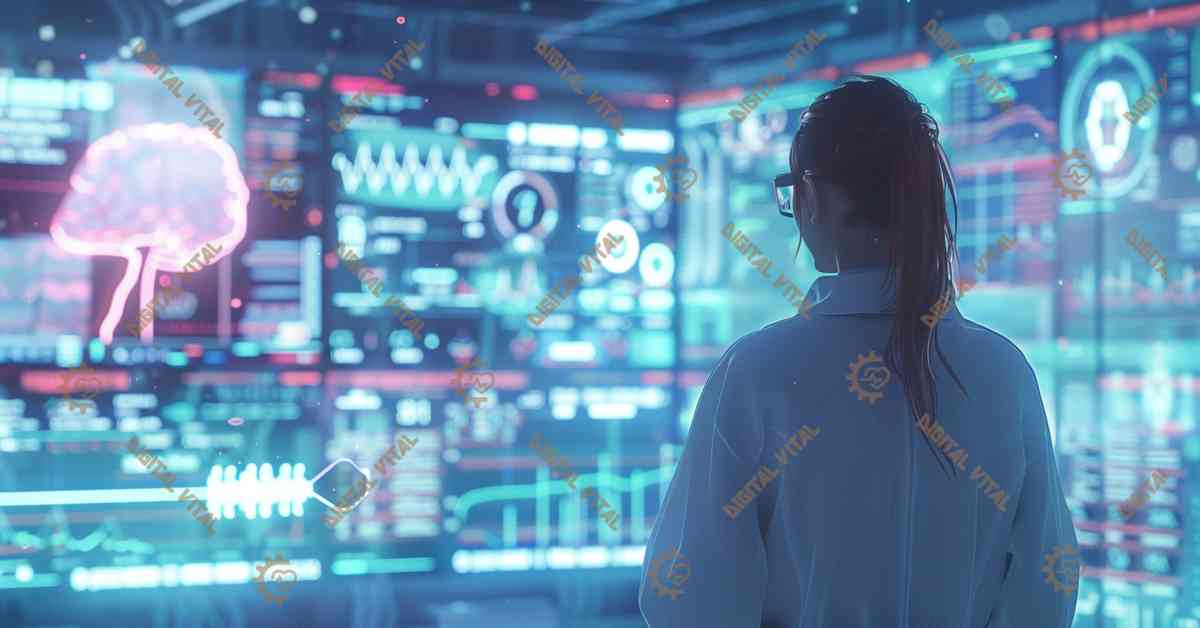
Challenges in 3D Reconstruction
The shift from 2D to 3D representations in medical imaging brought with it several unique particular challenges. From data collection to final modeling, every step of the 3D reconstruction process has unique procedural and technical complexities. To fully utilize 3D imaging’s diagnostic potential, these challenges must be addressed and overcome. We will go over a few of these major challenges in the sections that follow, providing insight into the real-world difficulties faced by experts in the field of medical image processing.
Various complexities involve in 3D reconstruction, and they have significant effects on the technical procedure as well as clinical results. Among the challenges faced by creating accurate 3D models are data integrity, variation management in resolution, and computational efficiency. It is essential that these challenges be tackled with an understanding of how they will affect the 3D reconstruction procedure and the following medical assessments that depend on it.
Data Quality
Accurate 3D reconstruction is based on the foundation of excellent data acquisition. But there are a number of factors that can make this hard to achieve. Images that are blurry or lacking in detail can be caused by patient movement, the natural movement of organs, and the technological limitations of scanners. These problems could obscure crucial details needed to create a high-fidelity 3D model.
Data Incompleteness
In some cases, data collected may not cover all essential anatomical regions or may do so with insufficient resolution. This is especially true while trying to reduce the patient’s radiation exposure or in areas that are challenging to scan. Incomplete data sets can cause Gaps in the 3D reconstruction, making it less reliable for therapeutic and diagnostic applications.
Noise and Artifacts
Significant challenges are presented by noise, which are random variations in image intensity, and artifacts, which are structural deformities not found in the genuine anatomy. They can be introduced by the imaging equipment or patient-related parameters, such as metal implants causing streak artifacts in CT images. These can complicate accurate reconstruction by masking real anatomical characteristics and introducing false positives.
Resolution Discrepancies
Inconsistencies in the 3D model may result from discrepancies in image resolution. Detailed reconstructions can be produced from high-resolution images, but obtaining them can be time-consuming and even dangerous because of increased radiation exposure. On the other hand, while low-resolution images are safer and faster to obtain can, but the result could be an oversimplified model lack critical details.
Computational Demands
3D reconstruction is computationally intensive. Reconstructing complex structures from large datasets might demand a significant amount of computational resources. The computational resources that are accessible to facilities can present challenges, which may limit their capacity to carry out high-resolution reconstructions or increase processing times.
Contrast and Intensity Variations
Variations in the contrast and intensity levels of medical images can have a direct effect on how accurate the 3D model is. Inconsistent intensity values across slices or modalities can result in a model with uneven quality, with some regions lacking definition and others well-defined. This could potentially lead to misinterpretation of anatomical structures.
Segmentation Accuracy
Segmentation, the process of delineating structures within an image, is essential for 3D reconstruction. Since the model’s accuracy depends on the precise definition of structure’s boundaries, segmentation errors can result in serious inaccuracies in the model. Dealing with pathologies that lack distinct boundaries makes this work considerably challenging.
Software Limitations
There could be limitations with the reconstruction software that affect the final model. These can include inadequate tools for correcting data errors, the incapacity to handle large data sets effectively, and lack of support for the latest imaging modalities. Software that is unable to handle the demands of complex reconstructions may significantly reduce the output’s quality.
Operator Expertise
The skill level of the operator performing the reconstruction can be a challenge. Inexperienced operators may not optimize parameter settings or may misinterpret the data, leading to suboptimal reconstructions. Operator expertise is essential to navigate the sophisticated software and hardware used in the reconstruction process.
A multifaceted strategy is needed to address these issues, including advancements in imaging technology, improved computational algorithms, and rigorous training for operators. In order to maximize the benefits of 3D medical imaging in patient care, these obstacles must be overcome.
Factors Affecting 3D Reconstruction
Many factors affect 3D reconstruction in medical imaging, which might affect the final model’s accuracy and usefulness. Understanding these factors is essential for improving reconstruction quality and for the effective use of 3D models in clinical practice.
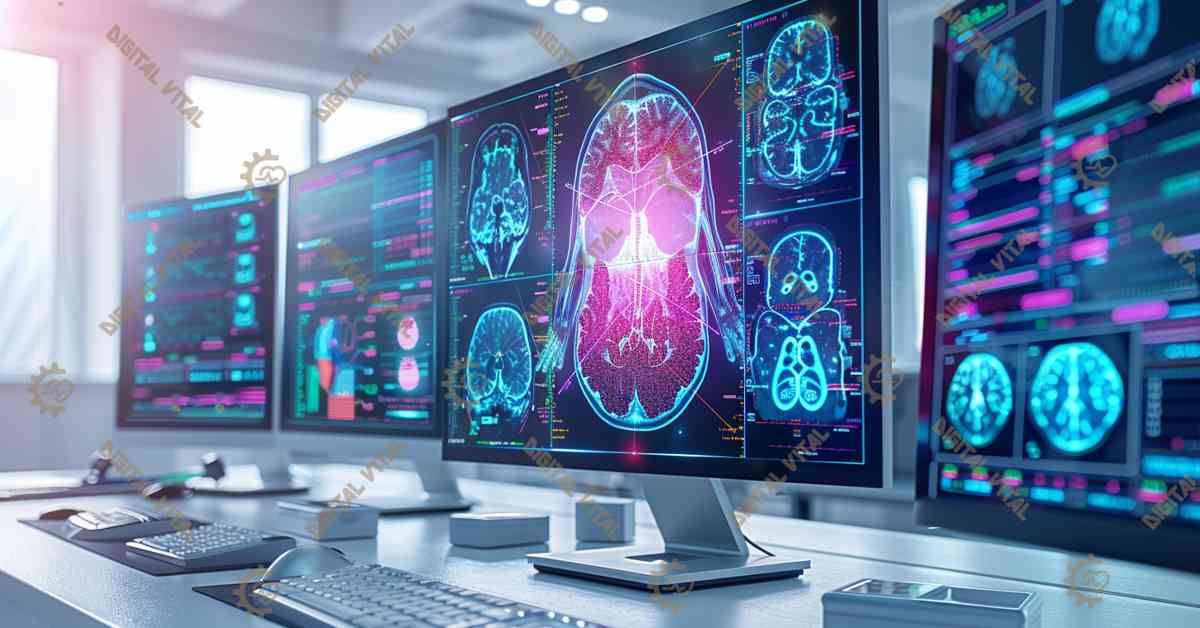
Measurement and Quantitative Factors
Accurate measurements are the cornerstone of precise 3D reconstructions. Quantitative factors such as the dimensions, volume, and density of anatomical structures must be captured accurately by imaging devices. Variations or inaccuracies in these measurements might result in models that are inaccurate representations of the real anatomy, which may have an impact on diagnostic decisions and treatment plans.
Geometrical Considerations
The geometry of the collected data plays a significant role in reconstruction. The spatial relationship between different anatomical elements should be preserved during the imaging and reconstruction procedure. Deviations in geometrical integrity can result from improper imaging techniques or misalignment during image acquisition, leading to a distorted 3D representation.
Accuracy of Imaging Devices
The precision of the imaging devices themselves is a crucial factor. Calibration and maintenance of these devices ensure that the data collected are as close to the patient’s actual anatomy as possible. Any discrepancy in the device’s performance can introduce errors, which are then propagated through the reconstruction process.
Quality of Measurement
The quality of the data, which can be influenced by the resolution, signal-to-noise ratio, and contrast of the images, affects the reconstruction outcome. Accurately recognizing subtle anatomical features and pathologies in the images requires high-quality measurements.
Data Types and Sources
Different imaging modalities provide various types of data, each with its own advantages and limitations. For example, CT scans offer excellent bone detail but less soft tissue contrast, whereas MRI provides high soft tissue contrast. As a result, the type of data used can affect the reconstructed model’s accuracy and level of detail.
Computational Algorithms
The algorithms used to process and reconstruct the images can significantly affect the final model. The choice of algorithm determines how data are interpolated, how noise is managed, and how edges and surfaces are rendered. Selecting the most appropriate algorithm for the data and the clinical requirement is crucial for an accurate reconstruction.
Practically, by carefully considering these factors, professionals in medical imaging can enhance the accuracy and reliability of 3D reconstructions. Each factor contributes to the overall quality of the model and, therefore, to the reliability of the information that clinicians derive from them. Addressing these factors is a step toward more accurate and clinically useful 3D medical imaging.
Algorithmic Considerations in 3D Medical Imaging Reconstruction
The effectiveness of 3D reconstruction in medical imaging largely hinges on the choice and implementation of algorithms. This section delves into several key algorithmic considerations that significantly influence the reconstruction process.
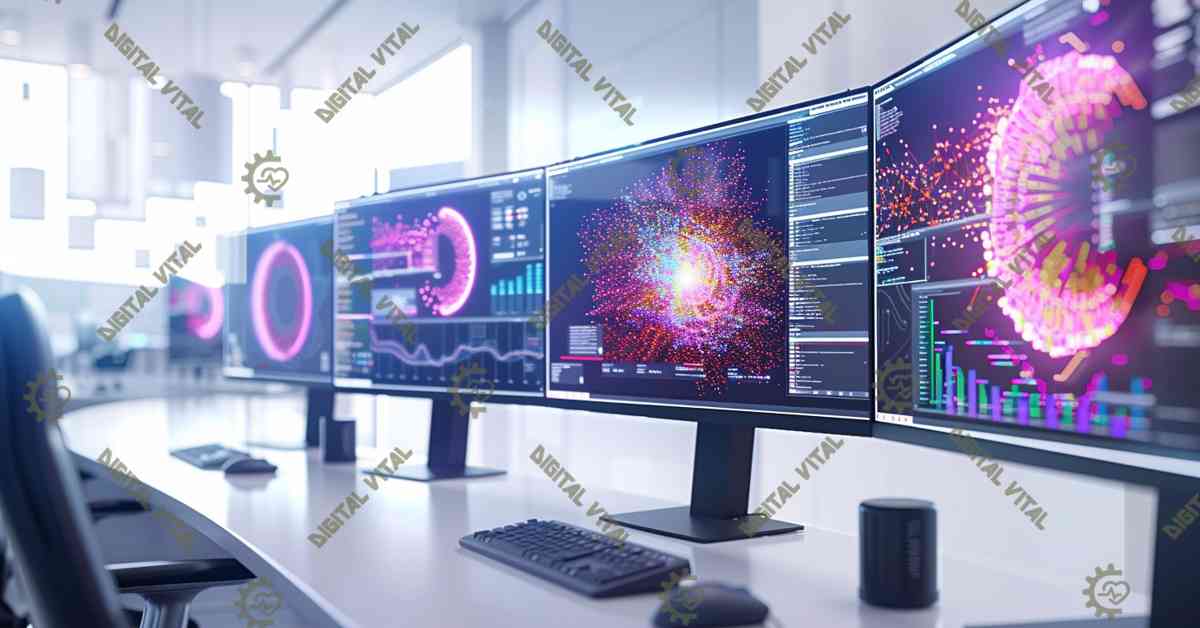
Algorithm Selection Based on Data Type
Different imaging modalities yield diverse types of data, each requiring tailored algorithms for optimal reconstruction. For instance, algorithms used for CT data, which provide high-density resolution, differ from those suitable for MRI data, known for their soft tissue differentiation. The selection of the right algorithm must align with the specific characteristics of the data being processed.
Noise Reduction and Image Enhancement
Algorithms play a crucial role in minimizing noise and enhancing image quality. Techniques like filtering and smoothing are employed to reduce random noise in the images, while edge enhancement algorithms can improve the clarity of structural boundaries. Choosing the right balance of noise reduction and image enhancement is critical to maintain the integrity of anatomical details.
Segmentation Algorithms
Segmentation, the process of separating different anatomical structures within the image, is a vital step in 3D reconstruction. Algorithms vary in their approach to segmentation, with some focusing on texture, others on color intensity, and others on geometrical features. The effectiveness of segmentation directly impacts the accuracy of the 3D model.
Computational Efficiency
The computational demand of 3D reconstruction algorithms is another important consideration. Some algorithms, while providing high accuracy, may require significant processing power and time. Facilities must consider their computational capacity when choosing algorithms, especially when dealing with large datasets or requiring quick turnaround times.
Handling Data Incompleteness and Misalignments
Reconstruction algorithms must also account for incomplete data or misalignments in the imaging slices. Advanced algorithms can interpolate missing data or correct misalignments, but the effectiveness of these corrections varies. The choice of algorithm in such scenarios can greatly affect the fidelity of the reconstructed model.
Integration with Clinical Workflow
Finally, the integration of these algorithms into the clinical workflow is essential. Algorithms should be user-friendly and compatible with existing medical imaging systems. Their outputs must be interpretable and actionable for medical professionals, ensuring that the reconstructed models are not only accurate but also clinically relevant.
In sum, the choice and application of algorithms in 3D medical imaging reconstruction are complex but critical decisions. Each algorithm brings its own set of strengths and limitations, impacting the quality, accuracy, and usability of the reconstructed models. As technology advances, ongoing research and development in this area will continue to refine these algorithms, enhancing the role of 3D reconstruction in medical diagnostics and treatment planning.
Conclusion
This article’s investigation of 3D medical imaging reconstruction has brought to light a number of crucial challenges and concerns for the medical imaging sector. Anything from the difficulties of data acquisition to the complexities of algorithm selection affects how accurate and useful 3D reconstructed images are. Leveraging the full potential of 3D imaging in medical diagnostics and treatment planning requires successfully navigating these obstacles.
Achieving precise 3D reconstructions requires accuracy at every step of the process. It requires careful attention to detail in all aspects, including data processing and measurement, handling geometric and resolution discrepancies, and applying complex computational algorithms. In addition, the integration of these technical procedures into clinical workflows emphasizes how important it is to maintain a balance between technical accuracy and practical applicability.
As we move forward, the field of medical imaging stands on the brink of significant advancements. Continued research, development, and collaboration across disciplines are essential to overcome the existing obstacles. The goal is clear: to refine 3D reconstruction processes to a level where they become indispensable tools in the hands of medical professionals, offering clearer insights into patient anatomy and pathologies.
In summary, despite their scope, the challenges in 3D medical imaging reconstruction offer a path forward for further development and provide a roadmap for future innovation. Addressing these challenges head-on will not only enhance the technical capabilities of 3D reconstructions but will also pave the way for more accurate diagnoses, better patient outcomes, and the continued evolution of medical imaging technology.
References
- Bushberg, Jerrold T., et al. The Essential Physics of Medical Imaging. 3rd ed., Lippincott Williams & Wilkins, 2011.
- Suetens, Paul. Fundamentals of Medical Imaging. 2nd ed., Cambridge University Press, 2009.
- Peters, Terry M., and Kevin Cleary. Image-Guided Interventions: Technology and Applications. Springer, 2008. Book
- Herman, Gabor T. Fundamentals of Computerized Tomography: Image Reconstruction from Projections. 2nd ed., Springer, 2009.
- McRobbie, Donald W., et al. MRI from Picture to Proton. 2nd ed., Cambridge University Press, 2006.
Further Reading
- Bankman, Isaac N. (Ed.). Handbook of Medical Imaging: Processing and Analysis. Academic Press, 2000.
- Prince, Jerry L., and Jonathan M. Links. Medical Imaging Signals and Systems. 2nd ed., Pearson, 2014.
- Webb, Steve. The Physics of Medical Imaging. Institute of Physics Publishing, 2012.
- Gonzalez, Rafael C., and Richard E. Woods. Digital Image Processing. 3rd ed., Pearson, 2008.
- Seeram, Euclid. Computed Tomography: Physical Principles, Clinical Applications, and Quality Control. 5th ed., Elsevier Health Sciences, 2022.
For small businesses, employees, job seekers, and students, DigitalVital offers comprehensive services to help you navigate and thrive. Whether you need a Business strategy consultancy, publishing support, CV review, or specialized further education, we are here to assist you.
Explore other articles on our platform to stay updated on the latest trends and insights in Image Processing and related fields.