In the fast-evolving landscape of manufacturing, integrating artificial intelligence (AI) and machine learning (ML) is proving to be a game-changer. These technologies are not only enhancing productivity but also significantly improving worker safety. As the manufacturing sector transitions towards Industry 5.0, a human-centric approach becomes crucial. This article delves into the role of AI and ML in manufacturing, highlighting their impact on safety and productivity, and discussing the ethical considerations essential for their deployment.
Key phrases and keywords: human-centric AI, manufacturing productivity, AI worker safety, ethical AI in manufacturing, machine learning in industry
Table of Contents
The Role of AI and Machine Learning in Enhancing Worker Safety
AI-Driven Safety Measures
Artificial intelligence and machine learning technologies are revolutionizing worker safety in manufacturing. AI-powered systems can predict potential hazards by analyzing data from sensors and cameras placed throughout the workplace. These systems utilize sophisticated algorithms to detect unsafe conditions, such as equipment malfunctions, hazardous material spills, or even improper worker behavior, in real-time. For instance, machine vision technologies can identify when a worker is too close to dangerous machinery, triggering automatic shutdowns to prevent accidents. According to a study by the International Labour Organization, such proactive measures can reduce workplace accidents by up to 30%.
Moreover, wearable devices embedded with AI capabilities are increasingly used to monitor workers’ health and safety. These devices can track vital signs, fatigue levels, and environmental conditions, alerting both the worker and management to potential health risks. For example, smart helmets equipped with sensors can monitor brain activity and detect signs of fatigue, prompting rest periods to prevent accidents caused by drowsiness. A report by ABI Research predicts that by 2025, the market for wearable devices in the industrial sector will reach $54 billion, highlighting their growing significance.
In addition to wearables, AI-driven safety measures extend to environmental monitoring. Advanced AI systems can analyze air quality, temperature, and humidity levels in real-time to ensure that the working conditions remain within safe limits. In industries dealing with hazardous materials, AI can detect leaks or spills, triggering immediate containment protocols and alerting emergency response teams. For example, in the chemical manufacturing industry, AI systems are used to monitor storage tanks for potential leaks, reducing the risk of catastrophic accidents.
By leveraging AI, manufacturers can create a safer work environment, proactively addressing issues before they result in injuries. The implementation of AI-driven safety measures not only protects workers but also enhances overall operational efficiency, reducing downtime caused by accidents and improving compliance with safety regulations. As the technology evolves, the integration of AI in safety protocols will continue to advance, providing increasingly sophisticated tools to ensure worker safety.
Machine Learning for Predictive Maintenance
Machine learning (ML) enhances worker safety by enabling predictive maintenance, a critical aspect of modern manufacturing. Traditionally, equipment failures have posed significant risks to workers, often leading to accidents, injuries, and costly downtime. ML algorithms can analyze historical and real-time data from machinery to predict when a component is likely to fail, allowing for timely maintenance and reducing the risk of unexpected breakdowns.
Predictive maintenance involves collecting data from various sensors embedded in machinery, such as vibration, temperature, and pressure sensors. This data is then processed by Machine Learning algorithms to identify patterns and anomalies that indicate potential issues. For example, an ML model can detect unusual vibrations in a motor, which may signify an impending failure. By addressing the problem before it escalates, manufacturers can prevent accidents and ensure continuous operation.
The benefits of predictive maintenance are well-documented. According to a study by Deloitte, predictive maintenance can reduce maintenance costs by 20% and downtime by 50%. Companies like GE and Siemens have successfully implemented ML for predictive maintenance, demonstrating significant reductions in machinery-related accidents. GE’s Predix platform, for instance, uses advanced analytics to monitor industrial assets, predicting failures and optimizing maintenance schedules. This not only enhances worker safety but also extends the lifespan of equipment, ensuring a safer and more reliable work environment.
Furthermore, predictive maintenance contributes to sustainability efforts by reducing waste. Timely maintenance prevents unnecessary wear and tear, minimizing the need for replacement parts and reducing the environmental impact of manufacturing processes. A report by McKinsey & Company highlights that predictive maintenance can reduce CO2 emissions by up to 10% by optimizing energy consumption and minimizing equipment failures.
In addition to its safety benefits, predictive maintenance also plays a crucial role in improving overall productivity. By preventing unexpected breakdowns, manufacturers can maintain a steady production flow, meeting delivery schedules and customer demands more effectively. The integration of IoT devices with ML algorithms enables real-time monitoring and analysis, providing actionable insights that help manufacturers make informed decisions.
In conclusion, the application of machine learning in predictive maintenance is transforming the manufacturing industry. By predicting equipment failures and enabling timely interventions, ML enhances worker safety, reduces costs, and improves operational efficiency. As technology continues to advance, the adoption of predictive maintenance will become increasingly prevalent, driving further improvements in safety and productivity across the manufacturing sector.
Boosting Productivity with AI and Machine Learning
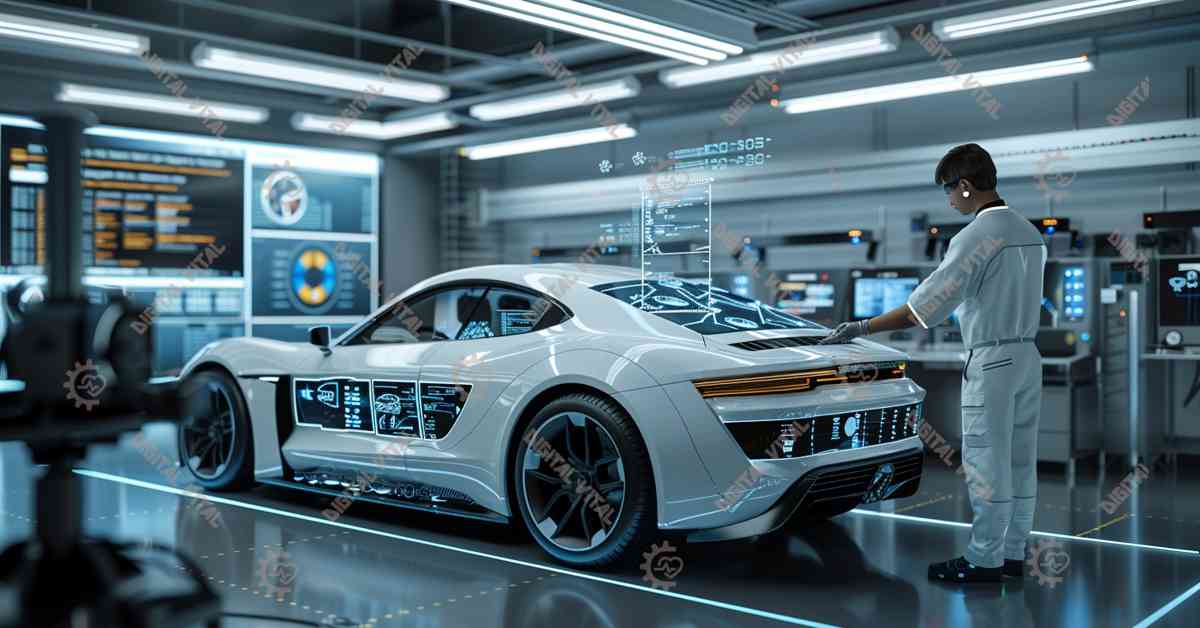
Optimizing Manufacturing Processes
AI and ML are pivotal in optimizing manufacturing processes, leading to enhanced productivity. These technologies can analyze vast amounts of data from production lines to identify inefficiencies and suggest improvements. For example, AI algorithms can optimize supply chain operations by predicting demand and adjusting inventory levels accordingly. This ensures that materials are available when needed, reducing downtime and increasing overall efficiency.
AI-driven analytics are transforming the traditional manufacturing landscape by enabling real-time decision-making. For instance, IBM’s Watson AI platform leverages machine learning to monitor and analyze production data, providing actionable insights that help streamline operations. According to a report by McKinsey & Company, companies that have implemented AI-driven analytics have seen a 15-20% increase in production efficiency. This is achieved by reducing bottlenecks, optimizing machine usage, and improving workflow management.
Furthermore, AI-powered robots and cobots (collaborative robots) are revolutionizing the manufacturing floor. These machines can work alongside human workers, handling repetitive and physically demanding tasks. Cobots, such as those produced by Universal Robots, are designed to be safe and intuitive to use, making it easier for workers to adapt to them. This collaboration not only boosts productivity but also reduces the physical strain on workers, allowing them to focus on more complex and creative tasks. A study by the International Federation of Robotics found that the global market for cobots is expected to grow by 20% annually, underscoring their increasing importance in modern manufacturing.
Additionally, Human-Centric AI can enhance the flexibility of manufacturing processes. For example, in the textile industry, AI-driven systems can adjust production parameters on the fly to accommodate changes in design or material, significantly reducing the time and cost associated with retooling. This level of agility allows manufacturers to respond more swiftly to market demands and customize products to meet specific customer needs. The integration of AI and ML in manufacturing processes not only improves efficiency but also drives innovation, enabling companies to maintain a competitive edge in a rapidly evolving market.
Enhancing Quality Control
Machine learning plays a crucial role in quality control, another key aspect of productivity in manufacturing. ML algorithms can analyze product data to detect defects and inconsistencies that might be missed by human inspectors. Automated quality control systems powered by AI can perform inspections at a much faster rate, ensuring that only high-quality products reach the market.
For instance, in the automotive industry, AI-driven inspection systems can detect minute flaws in car parts, ensuring higher safety standards and reducing the need for costly recalls. Companies like Tesla and BMW utilize AI-based quality control systems to ensure that every vehicle component meets stringent safety and quality standards. A study by PwC revealed that AI-driven quality control systems could reduce defect rates by up to 90%, leading to significant cost savings and improved product reliability.
In the electronics manufacturing industry, companies such as Foxconn use AI to inspect circuit boards for defects. Machine learning algorithms are trained to recognize patterns that indicate faulty components or assembly errors, which are then flagged for further inspection. This process not only speeds up the inspection process but also increases accuracy, reducing the likelihood of defective products reaching consumers.
Moreover, AI-powered quality control systems can provide valuable data for continuous improvement. By analyzing trends and patterns in defect data, manufacturers can identify root causes and implement corrective actions to prevent recurrence. This proactive approach to quality management leads to a cycle of continuous improvement, enhancing overall product quality and customer satisfaction. For example, a report by Deloitte highlights that AI-driven quality control can reduce warranty costs by up to 20%, as fewer defective products are shipped to customers.
By improving quality control, manufacturers can maintain high standards, minimize waste, and boost overall productivity. The use of AI and ML in quality control not only enhances the reliability of products but also contributes to the sustainability of manufacturing processes by reducing material waste and energy consumption. As these technologies continue to evolve, their impact on quality control will become even more profound, driving further advancements in manufacturing efficiency and product excellence.
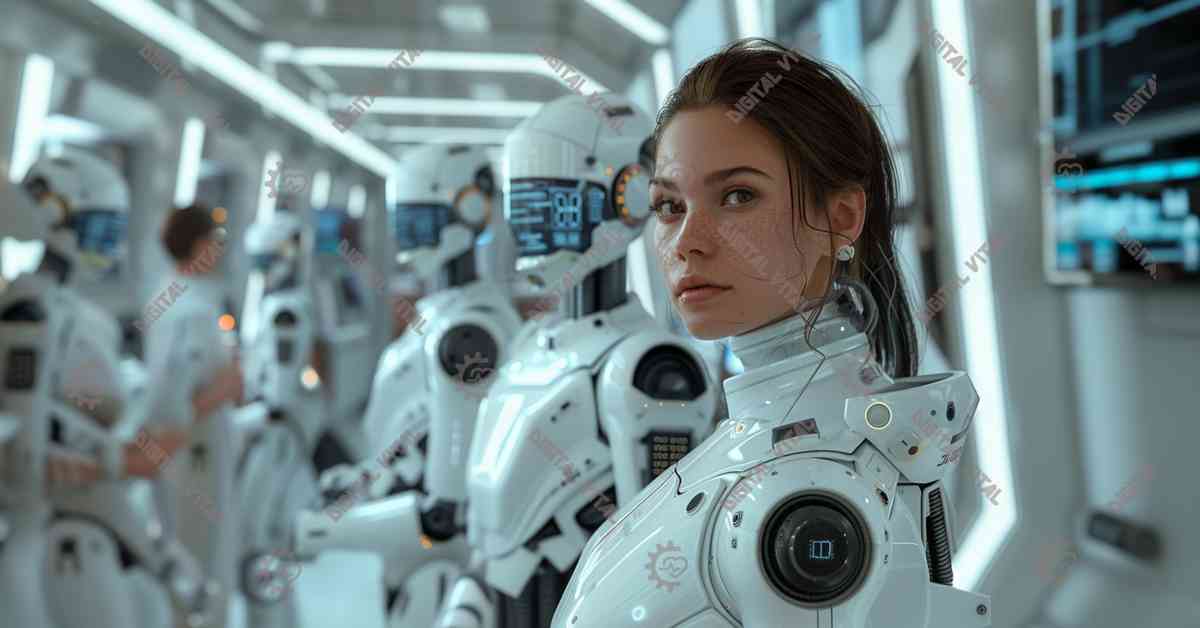
Ethical Considerations in Deploying AI in Industrial Settings
Ensuring Data Privacy and Security
While AI offers numerous benefits, its deployment raises significant ethical considerations, particularly regarding data privacy and security. AI systems rely on vast amounts of data, often collected from workers and manufacturing processes. Ensuring that this data is collected, stored, and used ethically is paramount. Companies must implement robust data protection measures to safeguard sensitive information and comply with regulations such as the General Data Protection Regulation (GDPR).
Data privacy is a critical concern in the era of Industry 5.0. AI systems require access to sensitive information, including employee health data, performance metrics, and operational details. Unauthorized access or misuse of this data can lead to severe consequences, including identity theft, financial loss, and reputational damage. To mitigate these risks, companies must adopt comprehensive cybersecurity frameworks. According to a report by Cybersecurity Ventures, cybercrime damages are expected to reach $10.5 trillion annually by 2025, underscoring the urgent need for robust security measures.
Transparency in data handling practices is also crucial. Workers should be informed about what data is being collected, how it is used, and the measures in place to protect their privacy. This includes implementing clear data usage policies and providing regular updates on data handling practices. For instance, companies like Siemens have adopted transparency frameworks that ensure employees are aware of data collection processes and their rights concerning personal data. Building trust through transparent and ethical data practices is essential for the successful integration of AI in manufacturing. The National Institute of Standards and Technology (NIST) provides guidelines for maintaining transparency and accountability in AI systems, which can serve as a valuable resource for companies.
Moreover, companies must invest in advanced encryption technologies to protect data at rest and in transit. Techniques such as differential privacy and federated learning can further enhance data security by allowing AI models to learn from data without directly accessing it. According to a study published in the Journal of Privacy and Confidentiality, these methods significantly reduce the risk of data breaches while maintaining the effectiveness of AI systems.
Addressing Bias in AI Algorithms
Another ethical concern is the potential for bias in AI algorithms. Bias can occur at various stages, from data collection to algorithm development, leading to unfair or discriminatory outcomes. In a manufacturing context, biased algorithms could impact worker safety and productivity, potentially favoring certain groups over others.
AI algorithms are only as unbiased as the data they are trained on. If the training data reflects existing biases, the AI system will likely perpetuate these biases. For example, an AI system trained on historical hiring data that favors certain demographics may inadvertently discriminate against other groups. This issue is particularly concerning in manufacturing, where biased algorithms could influence hiring decisions, performance evaluations, and even safety protocols. A study by MIT Media Lab found that facial recognition algorithms exhibited higher error rates for women and people of color, highlighting the pervasive nature of bias in AI systems.
To mitigate bias, it is important to ensure diversity in the teams developing AI technologies and to use diverse datasets for training algorithms. This includes actively involving individuals from various backgrounds and perspectives in the development process. For instance, Google’s AI ethics team emphasizes the importance of interdisciplinary collaboration and diverse representation in mitigating bias. By including a wide range of stakeholders, companies can identify potential biases and address them early in the development process.
Regular audits and evaluations of AI systems can help identify and rectify biases, ensuring fair and equitable outcomes for all workers. Companies should implement continuous monitoring frameworks to assess the performance of AI algorithms in real-world scenarios. Tools such as IBM’s AI Fairness 360 provide metrics for detecting and mitigating bias in AI models, offering valuable insights into algorithmic fairness. According to a report by the World Economic Forum, regular audits can reduce algorithmic bias by up to 30%, significantly enhancing the fairness and reliability of AI systems.
Additionally, companies should establish clear guidelines and policies for addressing bias in AI. This includes setting standards for data collection, algorithm development, and decision-making processes. The European Commission’s Ethics Guidelines for Trustworthy AI provide a comprehensive framework for ensuring ethical AI deployment, including principles for avoiding bias and ensuring transparency. By adhering to these guidelines, companies can foster a more inclusive and equitable manufacturing environment.
In conclusion, addressing bias in AI algorithms is crucial for the ethical deployment of AI in manufacturing. By ensuring diversity in development teams, using diverse datasets, conducting regular audits, and adhering to ethical guidelines, companies can mitigate bias and promote fair and equitable outcomes. As AI technologies continue to evolve, ongoing efforts to address bias will be essential in achieving a truly human-centric approach to AI in manufacturing.
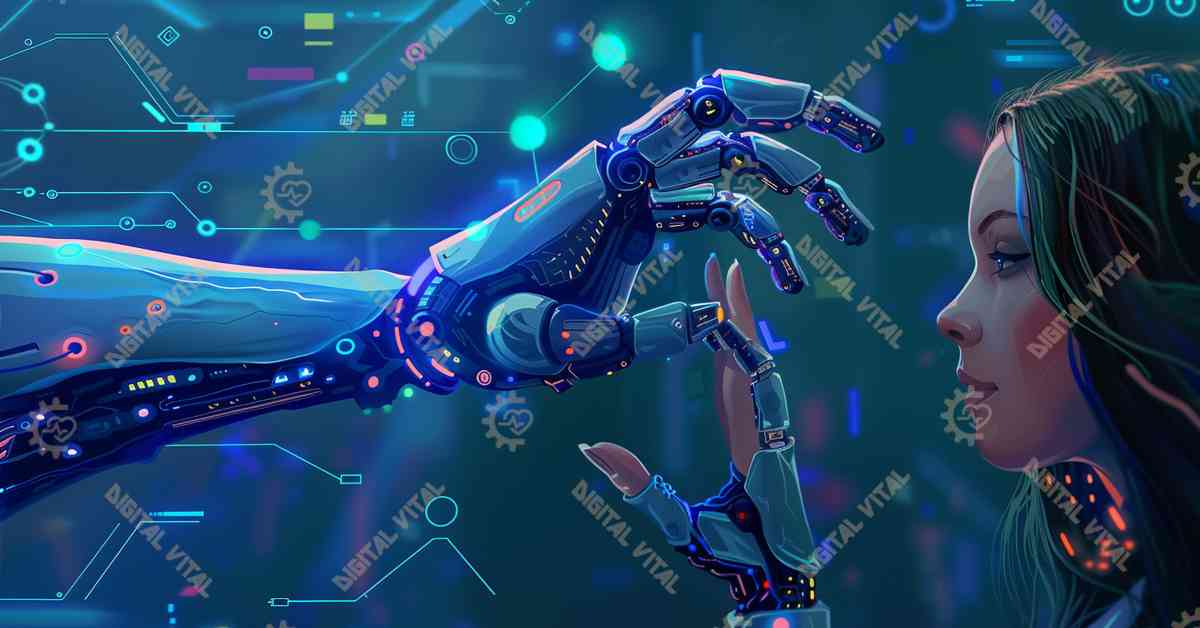
The Future of Human-Centric AI in Manufacturing
The integration of AI and machine learning in manufacturing is still evolving, with ongoing research and development aimed at making these technologies more effective and ethical. Future advancements in AI could lead to even more sophisticated safety systems, further enhancing worker protection. Additionally, as ethical AI practices become more ingrained, we can expect a more transparent and fair deployment of these technologies across the industry.
Collaborations between academia, industry, and policymakers will be crucial in shaping the future of human-centric AI in manufacturing. By prioritizing worker safety and productivity while addressing ethical concerns, the manufacturing sector can fully harness the potential of AI and ML, leading to a more sustainable and human-centric Industry 5.0.
Conclusion
The integration of AI and machine learning in manufacturing is revolutionizing the industry by enhancing both worker safety and productivity. AI-driven safety measures, such as real-time hazard detection and wearable health monitors, are making workplaces safer than ever before. Additionally, predictive maintenance powered by machine learning ensures equipment reliability, further safeguarding workers from unexpected machinery failures.
On the productivity front, AI and ML optimize manufacturing processes by identifying inefficiencies and suggesting improvements, while AI-powered robots and cobots alleviate workers from repetitive tasks, allowing them to focus on more complex and creative responsibilities. Machine learning also plays a crucial role in quality control, ensuring that only top-notch products reach the market, thus reducing waste and improving overall efficiency.
However, the deployment of AI in industrial settings brings forth significant ethical considerations. Ensuring data privacy and security, addressing algorithmic bias, and maintaining transparency in data handling are paramount for building trust and achieving fair outcomes. As the manufacturing sector continues to evolve towards Industry 5.0, it is essential to prioritize these ethical concerns to foster a human-centric approach that enhances worker well-being and productivity.
Looking ahead, the future of human-centric AI in manufacturing is promising. Continued advancements in AI technologies, coupled with robust ethical frameworks, will further elevate the industry’s capabilities. By fostering collaboration between academia, industry, and policymakers, the manufacturing sector can fully realize the potential of AI and ML, leading to a more sustainable, resilient, and human-centric future.
References
- Bortolini, M., Galizia, F. G., Mora, C., & Rosano, M. (2018). AI and IoT in Smart Manufacturing: A Review. Journal of Manufacturing Systems, 49, 39-52.
- Daugherty, P. R., & Wilson, H. J. (2018). Human + Machine: Reimagining Work in the Age of AI. Harvard Business Review Press.
- Gill, S. S., & Buyya, R. (2018). Machine Learning and AI in Manufacturing: The Next Industrial Revolution. IEEE Computer Society, 51(10), 67-70.
- Norman, D. A. (2023). Design for a Better World: Meaningful, Sustainable, Humanity-Centered. MIT Press.
- Turing, A. M. (1950). Computing Machinery and Intelligence. Mind, 59(236), 433-460.
- European Commission. (2024). ERA Industrial Technologies Roadmap on Human-Centric Research and Innovation for the Manufacturing Sector. Retrieved from European Commission Website.
By integrating cutting-edge AI and ML technologies while addressing ethical considerations, the manufacturing industry is set to achieve new heights in safety, productivity, and overall human-centricity.
For small businesses, employees, job seekers, and students, DigitalVital offers comprehensive services to help you navigate and thrive. Whether you need a Business strategy consultancy, publishing support, CV review, or specialized further education, we are here to assist you.
Explore other articles on our platform to stay updated on the latest trends and insights in Human-Centric AI and related fields.