Evidence based medicine (EBM) has revolutionized healthcare by emphasizing clinical decision-making based on rigorously tested and verified data. By combining research, clinical expertise, and patient preferences, EBM has become a key driver of healthcare improvements. In recent years, with the rise of digital technologies and advanced data processing tools, the integration of informatics, artificial intelligence (AI), and computer programming into evidence based medicine has transformed public health, treatment innovations, and multidisciplinary collaboration.
Zoe Amin-Akhlaghi, ZAMSTEC Academy of Science and Technology, Austria
Evidence based medicine, healthcare, public health, artificial intelligence, clinical decision-making, multidisciplinary collaboration, digital technology, patient outcomes, Qualitative Evidence Synthesis (QES)
Table of Contents
Introduction to Evidence Based Medicine
At its core, evidence based medicine is the conscientious, explicit, and judicious use of the best current evidence in making decisions about the care of individual patients. Evidence based medicine integrates the most current and relevant research data with clinical expertise, ensuring that medical decisions are informed by well-validated studies and not solely by intuition or traditional practices.
Historically, medical treatments were based on the expertise and intuition of physicians, often without a solid grounding in research. As medical science advanced, so did the demand for clinical decision-making that relied on proven outcomes from large, diverse studies. This shift toward evidence based medicine formalized in the early 1990s, thanks to pioneers like Dr. David Sackett, who helped structure a framework for integrating clinical knowledge with external research.
Today, evidence based medicine is an essential element in shaping public health strategies, advancing treatment methods, and ensuring optimal patient outcomes. Its use extends from individual care decisions to broad policy implementations that can impact entire populations.
The Historical Evolution of Evidence Based Medicine
The origins of evidence based medicine can be traced to early clinical trial reports in the mid-20th century. As medical research became more sophisticated, healthcare professionals began placing greater emphasis on using validated data to make treatment decisions. The establishment of organizations like the Cochrane Collaboration in 1993 was a critical turning point. This organization facilitated the widespread dissemination of systematic reviews and controlled trials, giving rise to a more structured and formal approach to evidence based medicine.
In the decades since, evidence based medicine has evolved dramatically. New technologies, data-processing systems, and innovations in the field of informatics have made it easier to aggregate, analyze, and synthesize large amounts of data. This shift has been instrumental in ensuring that evidence based medicine remains at the forefront of healthcare decision-making.
EBM’s Importance in Public Health Decision-Making
One of the most crucial areas where EBM has made a significant impact is in public health decision-making. In public health, decisions are often made on a larger scale, requiring data that spans a diverse array of populations, healthcare systems, and patient outcomes. Evidence based medicine ensures that these decisions are informed by the most reliable data available, leading to better health outcomes for populations at large.
An excellent example of this was during the COVID-19 pandemic, where public health policies related to lockdowns, vaccinations, and treatment protocols were all influenced by the best available evidence. Policymakers relied on evidence based medicine to analyze vaccine efficacy, monitor adverse effects, and determine the best course of action in minimizing the pandemic’s impact. This underscores how evidence based medicine plays a central role in shaping health policies that save lives and improve the quality of healthcare.
Additionally, Qualitative Evidence Synthesis (QES), a method used to systematically review qualitative research, is becoming an important tool in public health. It focuses on understanding patient experiences, healthcare delivery, and other qualitative aspects that traditional evidence based medicine may not capture fully. By integrating QES, public health policies can account for social, cultural, and behavioral factors, making them more effective and applicable across diverse populations.
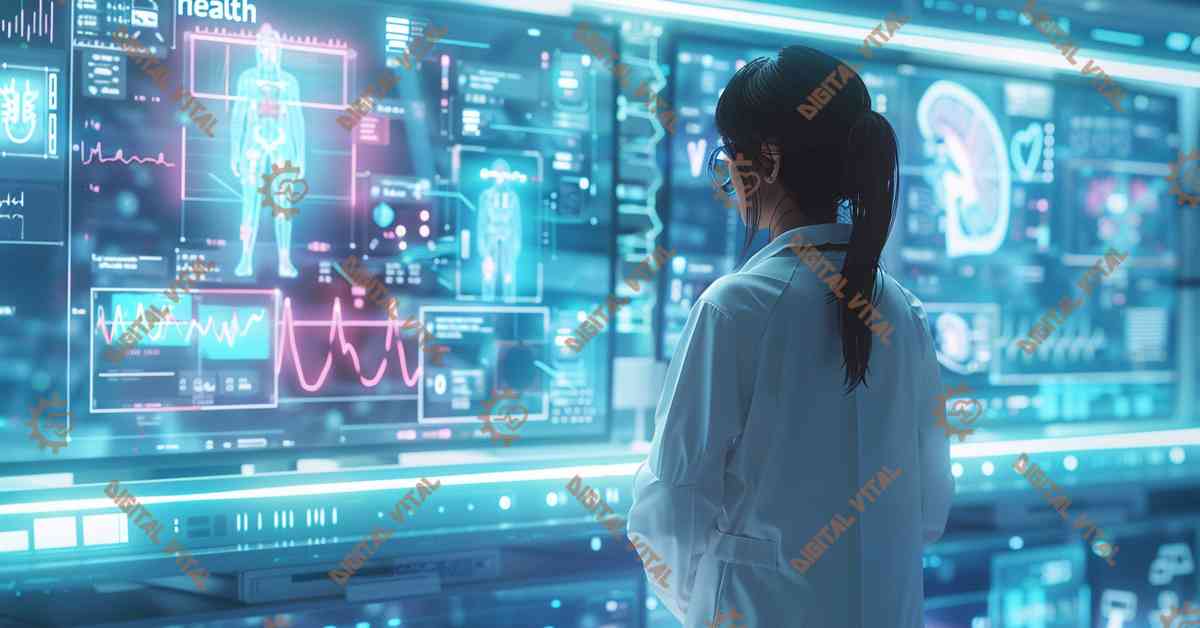
New Treatment Paradigms Through Evidence Based Medicine
One of the most significant contributions of EBM is its ability to drive the development of new treatment methods. EBM provides the framework necessary to evaluate new therapies, medications, and medical technologies through rigorous clinical trials and systematic reviews. This approach ensures that innovations in treatment are grounded in solid scientific evidence.
For instance, the introduction of immunotherapy in cancer treatment was once met with skepticism. However, after multiple large-scale studies demonstrated its effectiveness, it quickly became a staple of modern oncology. Similar breakthroughs have been seen in the management of chronic diseases like diabetes and heart disease, where evidence based medicine has helped optimize treatment protocols based on comprehensive data.
Moreover, precision medicine, which tailors treatment to an individual’s genetic profile, is another emerging area where evidence based medicine is instrumental. By using data from genomics and other biological markers, healthcare professionals can make more informed treatment decisions that are customized to each patient’s unique needs, leading to better outcomes.
A Multidisciplinary Approach to Evidence Based Medicine
What distinguishes evidence based medicine (EBM) today is its inherently multidisciplinary nature. EBM is no longer limited to healthcare professionals; it now involves a wide array of experts from different fields to harness the full potential of the data-driven healthcare landscape. The rise of digital technologies, artificial intelligence (AI), and large-scale data systems has broadened the expertise required to effectively implement evidence based medicine. Collaborating with professionals in fields such as data science, informatics, computer programming, and biostatistics is now essential for success. This multidisciplinary collaboration enhances the accuracy, efficiency, and applicability of EBM in improving patient care, public health policies, and healthcare innovation.
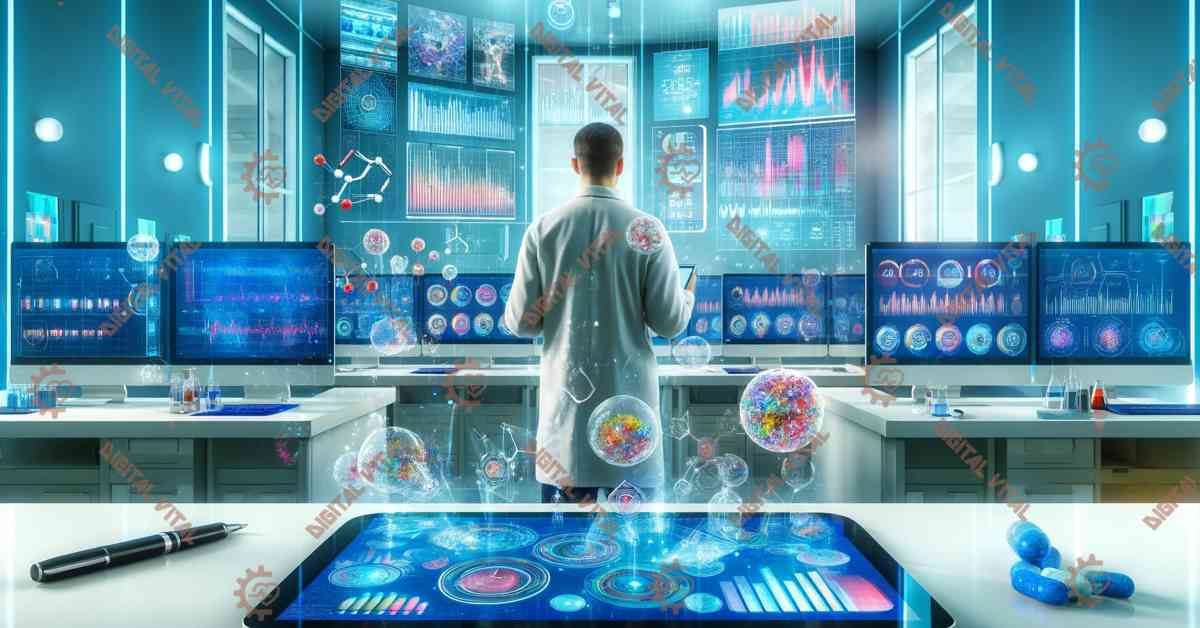
Informatics and Data Science
The sheer amount of data generated in modern healthcare—ranging from clinical trial data to real-world evidence (RWE) from electronic health records (EHRs)—poses significant challenges. Managing these vast datasets is one of the key aspects of modern evidence based medicine. Experts in informatics and data science are pivotal in this regard, as they help healthcare professionals aggregate, interpret, and utilize this data effectively.
In the United States alone, it is estimated that healthcare data will reach 2,314 exabytes by 2025, an exponential growth from just 153 exabytes in 2013 (EMC Digital Universe Study, 2014). This volume of data, often unstructured and scattered across multiple systems, requires advanced algorithms and machine learning models to process and extract meaningful insights.
For example, in oncology, the aggregation of genomic data, imaging results, and clinical outcomes requires advanced data analytics to determine the most effective treatment plans for cancer patients. The American Society of Clinical Oncology (ASCO) has implemented data science platforms that collate information from millions of cancer patients to provide real-time evidence on the effectiveness of treatments. Through data mining and machine learning, these platforms help identify new treatment pathways, predict patient responses, and improve clinical decision-making.
Informatics professionals also play a vital role in developing and maintaining clinical decision support systems (CDSS). These systems help clinicians apply evidence based guidelines by integrating patient data with the latest research, ensuring that care is personalized and grounded in solid evidence.
Artificial Intelligence (AI)
The role of artificial intelligence (AI) in evidence based medicine is expanding rapidly. AI has the unique ability to analyze vast datasets at speeds and scales unimaginable for humans. This allows for the identification of patterns and trends in health data, which can be used to inform diagnosis, predict outcomes, and recommend evidence-based treatments.
One of the most impactful areas of AI application in EBM is in medical imaging. AI-driven algorithms are now being used to detect diseases such as cancer and cardiovascular conditions earlier and more accurately than ever before. For instance, a 2019 study published in Nature demonstrated that AI systems could outperform radiologists in detecting breast cancer in mammograms, with an accuracy improvement of 11.5% (McKinney et al., 2020). This ability to harness AI for diagnostic purposes enhances the evidence base for treatment decisions, improving patient outcomes significantly.
Furthermore, AI is playing an increasing role in predictive analytics within EBM. For example, AI models can predict which patients are at higher risk of developing complications based on their medical histories, genetic profiles, and lifestyle data. These predictive insights allow clinicians to tailor interventions, using evidence based guidelines to prevent adverse outcomes before they occur.
Another prominent AI-driven application in EBM is natural language processing (NLP), which is being used to extract useful information from unstructured data sources such as research papers, medical notes, and clinical trial results. IBM Watson Health, for instance, uses AI and NLP to process vast amounts of clinical data, offering treatment recommendations based on the latest evidence available in scientific literature. This ability to instantly access and analyze evidence enhances decision-making at the bedside.
Computer Programming
Computer programming forms the backbone of the technologies that drive evidence based medicine today. Programmers and software engineers are responsible for developing decision-support tools that integrate patient data with evidence based clinical guidelines, making real-time healthcare decisions more efficient and accurate.
One key area where programming is crucial is in the development of Electronic Health Records (EHRs). EHR systems not only store patient data but are also capable of integrating with clinical decision support systems (CDSS), helping clinicians make evidence-based decisions at the point of care. By embedding evidence based algorithms directly into EHR platforms, computer programming ensures that clinicians have access to the latest evidence when making treatment decisions. This streamlines the process of applying research findings to clinical practice.
Moreover, machine learning and AI algorithms used in healthcare are built and maintained by programmers. These algorithms are used in various applications, from predictive analytics to diagnostic imaging. A well-designed decision-support system can process patient information and cross-reference it with the most current clinical guidelines to recommend personalized treatment options.
For example, platforms like Epic’s Best Practice Alerts use programming to issue automatic reminders to physicians about relevant evidence-based practices, such as reminding them of antibiotic stewardship guidelines or alerting them to new treatment options for chronic diseases.
Programming is also instrumental in developing healthcare apps that empower patients to participate in evidence based practices. Apps that track medication adherence, monitor symptoms, and provide evidence-based health tips have been shown to improve chronic disease management, leading to better patient outcomes.
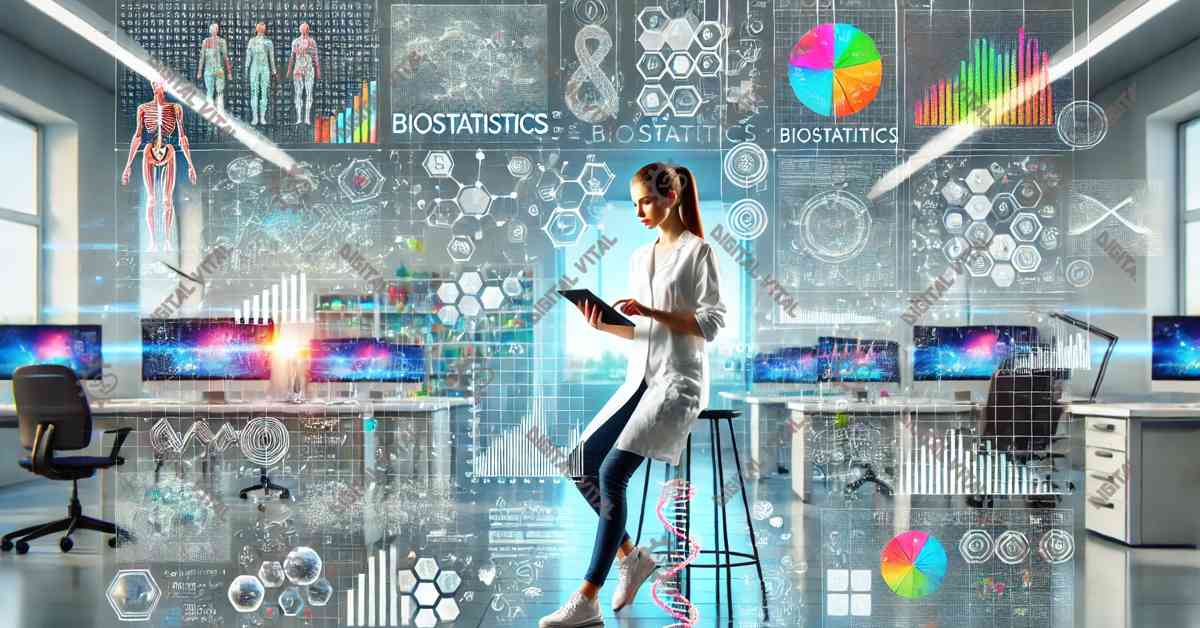
Statistics and Biostatistics
Biostatistics is fundamental to the very structure of evidence based medicine. Without robust statistical analysis, the results of clinical trials and observational studies would be difficult to interpret, and the conclusions drawn could be unreliable. Statisticians and biostatisticians play a key role in designing studies, analyzing data, and interpreting results to ensure that the findings are valid and applicable to broader populations.
In evidence based medicine, randomized controlled trials (RCTs) are considered the gold standard for evaluating the efficacy of treatments. However, conducting and analyzing RCTs involves complex statistical methodologies. Biostatisticians help ensure that the trials are well-designed, with proper randomization, blinding, and sample sizes that can produce statistically significant results. This ensures that the findings of the trial can be confidently applied to patient care.
For instance, a recent RCT published in The New England Journal of Medicine studied the effects of early intensive treatment for Type 2 diabetes. The study’s statistical analysis showed a 43% reduction in cardiovascular events among patients who received early aggressive treatment, providing robust evidence for changing treatment guidelines (Holman et al., 2018).
Moreover, meta-analyses, which synthesize data from multiple studies, rely heavily on advanced statistical techniques. By pooling data, statisticians can draw more generalized conclusions that strengthen the evidence base for particular treatments. These techniques help healthcare professionals make evidence based decisions that are grounded in a larger body of research, improving the reliability of clinical practice guidelines.
Lastly, biostatistics is critical for population health studies and public health decision-making. Large epidemiological studies, such as the Framingham Heart Study, have used statistical analysis to establish the risk factors for heart disease, influencing public health guidelines worldwide. Through statistical modeling, biostatisticians can predict health trends and evaluate the impact of interventions on entire populations, making them essential to evidence based public health policies.
The Role of Digital Technology in Evidence Based Medicine
Digital technology has significantly transformed evidence based medicine (EBM) by enabling healthcare professionals to access, process, and apply vast amounts of medical data in ways that were unimaginable a few decades ago. The integration of digital tools into EBM has improved the accuracy, speed, and accessibility of medical decisions, leading to more precise and personalized care. Technologies such as telemedicine, electronic health records (EHRs), mobile health applications, and artificial intelligence (AI) are redefining how evidence based medicine is practiced today.
Telemedicine and EHRs: Foundations for Digital EBM
Telemedicine has emerged as a crucial element in modern healthcare, especially in the context of evidence based medicine. It provides the ability to remotely diagnose, treat, and monitor patients, bridging gaps in healthcare access, especially for those in rural or underserved areas. According to a 2020 study published in The Lancet Digital Health, telemedicine saw a 154% increase in utilization during the COVID-19 pandemic, showcasing its vital role in delivering evidence-based care during times of crisis (Keesara et al., 2020).
Telemedicine platforms incorporate evidence-based protocols, allowing clinicians to deliver real-time medical advice and treatment plans based on the most current clinical guidelines. For example, telemedicine was used extensively to manage chronic conditions like diabetes and hypertension during the pandemic, ensuring that patients received evidence-based treatment while reducing the need for in-person visits. This was particularly important in protecting high-risk populations from exposure to COVID-19, while still providing them with essential healthcare.
Electronic health records (EHRs) are another cornerstone of digital technology in EBM. EHRs store vast amounts of patient data, including medical histories, lab results, and imaging studies, all of which can be easily accessed and analyzed. What makes EHRs indispensable in evidence based medicine is their ability to integrate with clinical decision support systems (CDSS). CDSS tools use patient data stored in EHRs to recommend treatments that align with the latest clinical guidelines, ensuring that healthcare decisions are rooted in solid, up-to-date evidence.
For example, Kaiser Permanente in the U.S. has implemented an EHR system that automatically alerts physicians to new evidence-based treatment recommendations, such as updated hypertension guidelines or reminders for cancer screenings. This real-time integration of evidence with patient care helps ensure that treatment is both timely and appropriate, leading to better patient outcomes.
Mobile Health Applications: Expanding Patient Participation in EBM
Mobile health applications, or mHealth apps, have also become an integral part of evidence based medicine by empowering patients to take a more active role in their healthcare. These apps allow patients to track their health data, such as blood pressure, blood sugar levels, and physical activity, which can be shared with healthcare providers for real-time monitoring.
A notable example is the mySugr app, which helps patients with diabetes manage their blood glucose levels. By tracking daily blood sugar readings and integrating with insulin pumps and continuous glucose monitors, mySugr provides patients with evidence-based feedback on how their diet, activity levels, and medication are affecting their health. A 2019 study published in Diabetes Therapy found that users of the app experienced a significant reduction in HbA1c levels, demonstrating how mobile health technology can effectively support evidence-based interventions (Charleer et al., 2019).
Moreover, mobile apps like AsthmaMD and Apple Health are leveraging evidence based medicine to create personalized health plans, track medication adherence, and provide reminders for routine screenings or vaccinations. These applications not only improve patient outcomes by adhering to evidence based guidelines but also promote patient-centered care, a crucial aspect of modern healthcare.
AI-Powered Diagnostics and Treatment Recommendations
One of the most significant advancements in digital technology within evidence based medicine is the application of artificial intelligence (AI). AI has the capability to analyze vast amounts of medical data quickly and efficiently, uncovering patterns and insights that might be missed by human analysis alone. This makes AI an invaluable tool for both diagnosis and treatment in EBM.
For example, IBM Watson Health uses AI to process patient records and match them with the most current clinical guidelines to make treatment recommendations. By leveraging natural language processing (NLP), Watson can sift through thousands of scientific papers, clinical trials, and guidelines to recommend personalized, evidence-based treatment options for patients. A study published in the Journal of Clinical Oncology showed that Watson Health achieved an accuracy rate of 90% in matching cancer treatment plans with the recommendations of oncologists, significantly improving the speed and precision of treatment decisions (Somashekhar et al., 2018).
Additionally, AI is revolutionizing diagnostic processes in fields like radiology and pathology. Deep learning algorithms can now detect diseases such as lung cancer, breast cancer, and diabetic retinopathy from medical images with accuracy rates that rival or even surpass those of experienced clinicians. A study published in Nature Medicine demonstrated that AI could detect lung cancer with a 94.6% accuracy rate, outperforming radiologists by a significant margin (Ardila et al., 2019). These findings underscore the role of AI in improving evidence-based diagnostics, leading to faster and more accurate treatment decisions.
Continuous Monitoring and Real-Time Data Collection
Another crucial advantage of digital technology in evidence based medicine is the ability to continuously monitor patient health. Wearable devices, such as smartwatches and fitness trackers, collect data on physical activity, heart rate, sleep patterns, and even oxygen levels. These devices can provide real-time health information to clinicians, allowing them to adjust treatments based on the most current data.
For instance, wearables like the Apple Watch can detect abnormal heart rhythms, prompting users to seek medical attention. A 2019 study published in The New England Journal of Medicine demonstrated that the Apple Watch was able to detect atrial fibrillation with 98.3% accuracy, providing real-time, evidence-based insights that can potentially save lives (Perez et al., 2019).
In chronic disease management, continuous glucose monitors (CGMs) allow healthcare providers to monitor a patient’s blood sugar levels in real-time, facilitating evidence-based adjustments to insulin therapy. CGMs have been shown to improve glucose control and reduce the risk of complications in patients with Type 1 diabetes. A study published in The Lancet found that CGMs reduced HbA1c levels by 0.5% and lowered the risk of severe hypoglycemia, highlighting the role of continuous monitoring in evidence based treatment (Battelino et al., 2019).
Qualitative Evidence Synthesis in EBM
While quantitative data is at the core of evidence based medicine, Qualitative Evidence Synthesis (QES) provides valuable insights into patient experiences, treatment barriers, and healthcare delivery. QES involves systematically reviewing qualitative research, such as patient interviews and case studies, to capture elements of healthcare that numbers alone may not convey.
For example, in chronic disease management, QES might reveal how cultural beliefs affect a patient’s willingness to adhere to treatment protocols. These findings can guide healthcare providers in developing interventions that are more aligned with the patient’s values and social context, leading to better compliance and outcomes.
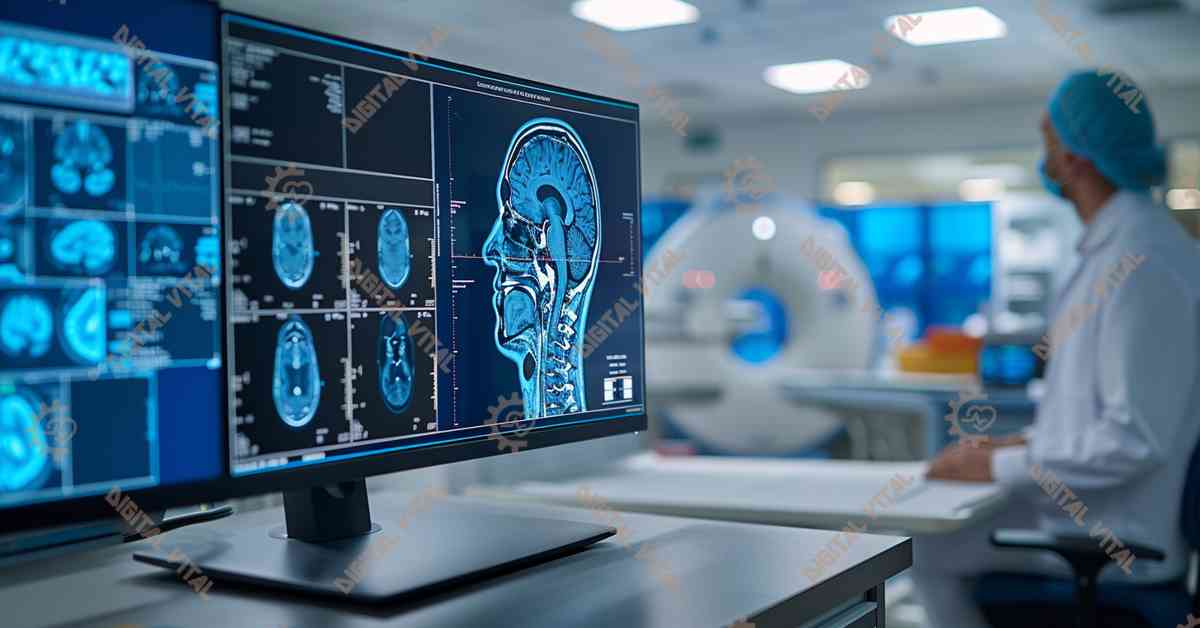
The Future of Evidence Based Medicine
The future of evidence based medicine lies at the intersection of healthcare and technology. With continued advancements in AI, data analytics, and digital health platforms, evidence based medicine will become even more precise and personalized. The ongoing collaboration between healthcare professionals and experts in other disciplines such as informatics, statistics, and computer programming will be critical in ensuring that EBM continues to evolve.
In addition, the integration of patient perspectives through Qualitative Evidence Synthesis ensures that future healthcare decisions will be not only data-driven but also patient-centered. This combination of quantitative data, qualitative insights, and cutting-edge technology promises a future where evidence based medicine will drive even greater improvements in healthcare quality and patient outcomes.
References
- Sackett, D. L., et al. (1996). “Evidence Based Medicine: What It Is and What It Isn’t.” BMJ, 312(7023), 71-72.
- Guyatt, G. H., et al. (2008). “Users’ Guides to the Medical Literature: A Manual for Evidence-Based Clinical Practice.” AMA Press.
- Cochrane Collaboration. (2022). “Cochrane Database of Systematic Reviews.” Retrieved from www.cochranelibrary.com
- Hoffman, T. C., et al. (2017). “The Role of Qualitative Evidence in Evidence Based Healthcare.” BMC Health Services Research, 17(1), 1-9.
- Watson Health. (2021). “AI-Powered Diagnostics and Decision Support.” IBM Research.
- Detmer, D., Bloomrosen, M., Raymond, B., & Tang, P. (2008). Integrated personal health records: Transformative tools for consumer-centric care. BMC Medical Informatics and Decision Making, 8, 45.
- McKinney, S. M., Sieniek, M., Godbole, V., Godwin, J., Antropova, N., Ashrafian, H., … & Suleyman, M. (2020). International evaluation of an AI system for breast cancer screening. Nature, 577(7788), 89-94.
- Holman, R. R., Paul, S. K., Bethel, M. A., Matthews, D. R., & Neil, H. A. W. (2018). 10-year follow-up of intensive glucose control in type 2 diabetes. The New England Journal of Medicine, 359(15), 1577-1589.
- Duffy, S. W., Vulkan, D., Cuckle, H., Parmar, D., Sheikh, S., Smith, R. A., & Evans, A. (2020). The impact of organised mammography service screening on breast carcinoma mortality in seven Swedish counties: A collaborative evaluation. Cancer, 126(23), 4877-4886.
- Rumsfeld, J. S., Joynt, K. E., Maddox, T. M. (2016). Big data analytics to improve cardiovascular care: Promise and challenges. Nature Reviews Cardiology, 13, 350-359.
- Keesara, S., Jonas, A., & Schulman, K. (2020). COVID-19 and health care’s digital revolution. The Lancet Digital Health, 2(8), e395-e396.
- Charleer, S., Mathieu, C., Nobels, F., De Block, C., Radermecker, R., Hermans, M. P., … & Veeze, H. J. (2019). Effect of the mySugr mobile health application on glycemic control in patients with type 1 diabetes: A randomized controlled trial. Diabetes Therapy, 10(1), 247-259.
- Somashekhar, S. P., Sepúlveda, M. J., Puglielli, S., Norden, A. D., Rauthan, A., Arun, K. V., … & Ethiraj, G. (2018). Watson for oncology and breast cancer treatment recommendations: Agreement with an expert multidisciplinary tumor board in India. JCO Clinical Cancer Informatics, (2), 1-8.
- Ardila, D., Kiraly, A. P., Bharadwaj, S., Choi, B., Reicher, J. J., Peng, L., & Corrado, G. (2019). End-to-end lung cancer screening with three-dimensional deep learning on low-dose chest computed tomography. Nature Medicine, 25(6), 954-961.
- Perez, M. V., Mahaffey, K. W., Hedlin, H., Rumsfeld, J. S., Garcia, A., Ferris, T., … & Turakhia, M. P. (2019). Large-scale assessment of a smartwatch to identify atrial fibrillation. The New England Journal of Medicine, 381(20), 1909-1917.
- Battelino, T., Danne, T., Bergenstal, R. M., Phillip, M., & Beck, R. (2019). Clinical targets for continuous glucose monitoring data interpretation: Recommendations from the international consensus on time in range. Diabetes Care, 42(8), 1593-1603.
For small businesses, employees, job seekers, and students, DigitalVital offers comprehensive services to help you navigate and thrive. Whether you need a Business strategy consultancy, publishing support, CV review, or specialized further education, we are here to assist you.
Explore other articles on our platform to stay updated on the latest trends and insights in Health care and related fields.